|
# Model Overview |
|
A pre-trained model for simultaneous segmentation and classification of nuclei within multi-tissue histology images based on CoNSeP data. The details of the model can be found in [1]. |
|
|
|
The model is trained to simultaneously segment and classify nuclei, and a two-stage training approach is utilized: |
|
|
|
- Initialize the model with pre-trained weights, and train the decoder only for 50 epochs. |
|
- Finetune all layers for another 50 epochs. |
|
|
|
There are two training modes in total. If "original" mode is specified, [270, 270] and [80, 80] are used for `patch_size` and `out_size` respectively. If "fast" mode is specified, [256, 256] and [164, 164] are used for `patch_size` and `out_size` respectively. The results shown below are based on the "fast" mode. |
|
|
|
In this bundle, the first stage is trained with pre-trained weights from some internal data. The [original author's repo](https://github.com/vqdang/hover_net) and [torchvison](https://pytorch.org/vision/stable/_modules/torchvision/models/resnet.html#ResNet18_Weights) also provide pre-trained weights but for non-commercial use. |
|
Each user is responsible for checking the content of models/datasets and the applicable licenses and determining if suitable for the intended use. |
|
|
|
If you want to train the first stage with pre-trained weights, just specify `--network_def#pretrained_url <your pretrain weights URL>` in the training command below, such as [ImageNet](https://download.pytorch.org/models/resnet18-f37072fd.pth). |
|
|
|
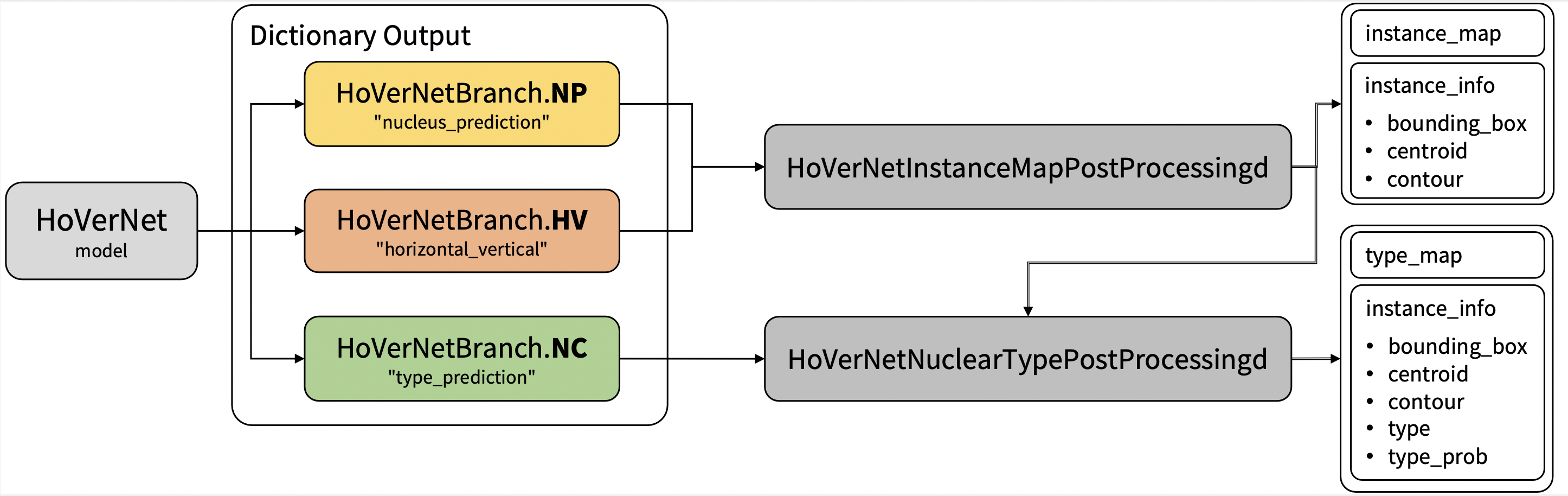 |
|
|
|
## Data |
|
The training data is from <https://warwick.ac.uk/fac/cross_fac/tia/data/hovernet/>. |
|
|
|
- Target: segment instance-level nuclei and classify the nuclei type |
|
- Task: Segmentation and classification |
|
- Modality: RGB images |
|
- Size: 41 image tiles (2009 patches) |
|
|
|
The provided labelled data was partitioned, based on the original split, into training (27 tiles) and testing (14 tiles) datasets. |
|
|
|
You can download the dataset by using this command: |
|
``` |
|
wget https://warwick.ac.uk/fac/cross_fac/tia/data/hovernet/consep_dataset.zip |
|
unzip consep_dataset.zip |
|
``` |
|
|
|
### Preprocessing |
|
|
|
After download the [datasets](https://warwick.ac.uk/fac/cross_fac/tia/data/hovernet/consep_dataset.zip), please run `scripts/prepare_patches.py` to prepare patches from tiles. Prepared patches are saved in `<your concep dataset path>`/Prepared. The implementation is referring to <https://github.com/vqdang/hover_net>. The command is like: |
|
|
|
``` |
|
python scripts/prepare_patches.py --root <your concep dataset path> |
|
``` |
|
|
|
## Training configuration |
|
This model utilized a two-stage approach. The training was performed with the following: |
|
|
|
- GPU: At least 24GB of GPU memory. |
|
- Actual Model Input: 256 x 256 |
|
- AMP: True |
|
- Optimizer: Adam |
|
- Learning Rate: 1e-4 |
|
- Loss: HoVerNetLoss |
|
- Dataset Manager: CacheDataset |
|
|
|
### Memory Consumption Warning |
|
|
|
If you face memory issues with CacheDataset, you can either switch to a regular Dataset class or lower the caching rate `cache_rate` in the configurations within range [0, 1] to minimize the System RAM requirements. |
|
|
|
## Input |
|
Input: RGB images |
|
|
|
## Output |
|
Output: a dictionary with the following keys: |
|
|
|
1. nucleus_prediction: predict whether or not a pixel belongs to the nuclei or background |
|
2. horizontal_vertical: predict the horizontal and vertical distances of nuclear pixels to their centres of mass |
|
3. type_prediction: predict the type of nucleus for each pixel |
|
|
|
## Performance |
|
The achieved metrics on the validation data are: |
|
|
|
Fast mode: |
|
- Binary Dice: 0.8291 |
|
- PQ: 0.4973 |
|
- F1d: 0.7417 |
|
|
|
Note: |
|
- Binary Dice is calculated based on the whole input. PQ and F1d were calculated from https://github.com/vqdang/hover_net#inference. |
|
- This bundle is non-deterministic because of the bilinear interpolation used in the network. Therefore, reproducing the training process may not get exactly the same performance. |
|
Please refer to https://pytorch.org/docs/stable/notes/randomness.html#reproducibility for more details about reproducibility. |
|
|
|
#### Training Loss and Dice |
|
stage1: |
|
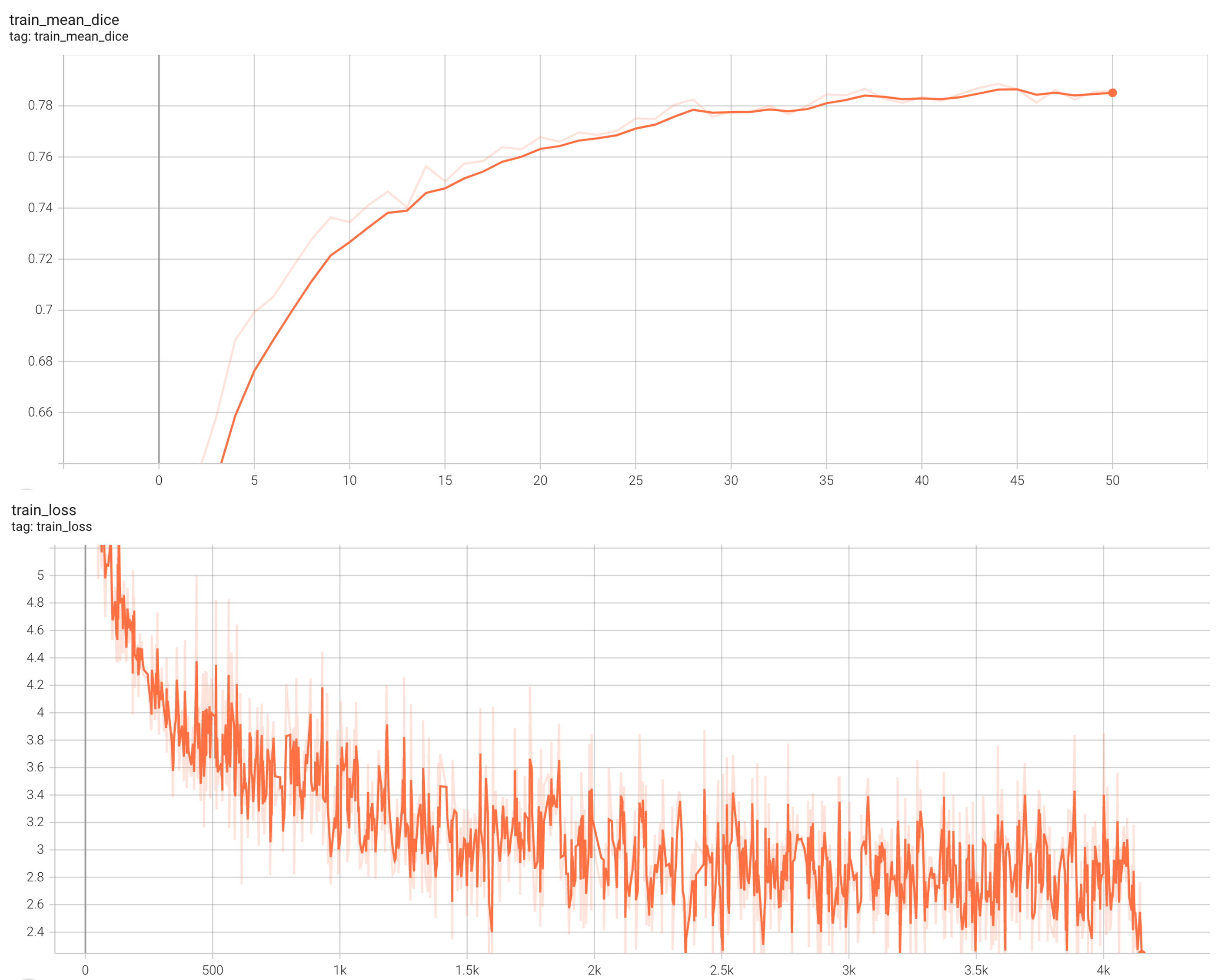 |
|
|
|
stage2: |
|
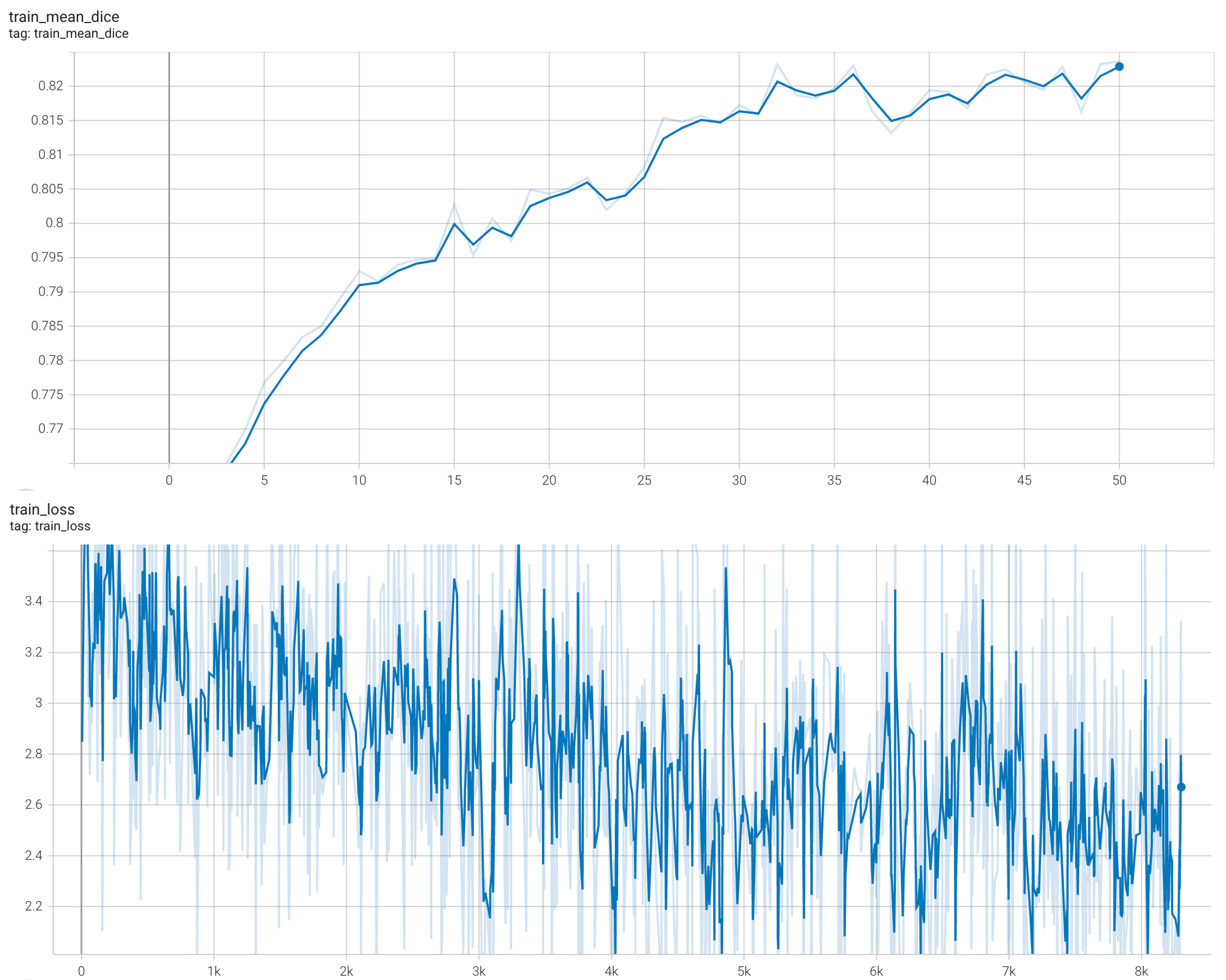 |
|
|
|
#### Validation Dice |
|
stage1: |
|
|
|
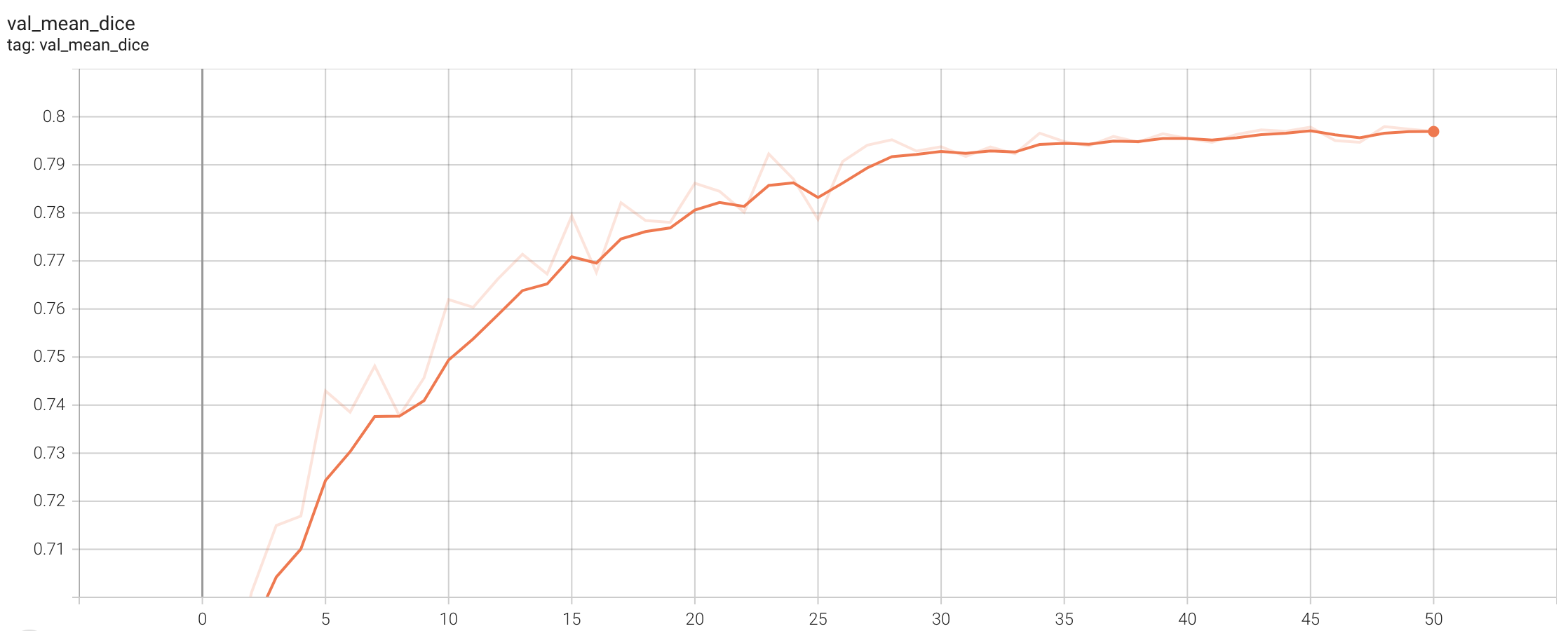 |
|
|
|
stage2: |
|
|
|
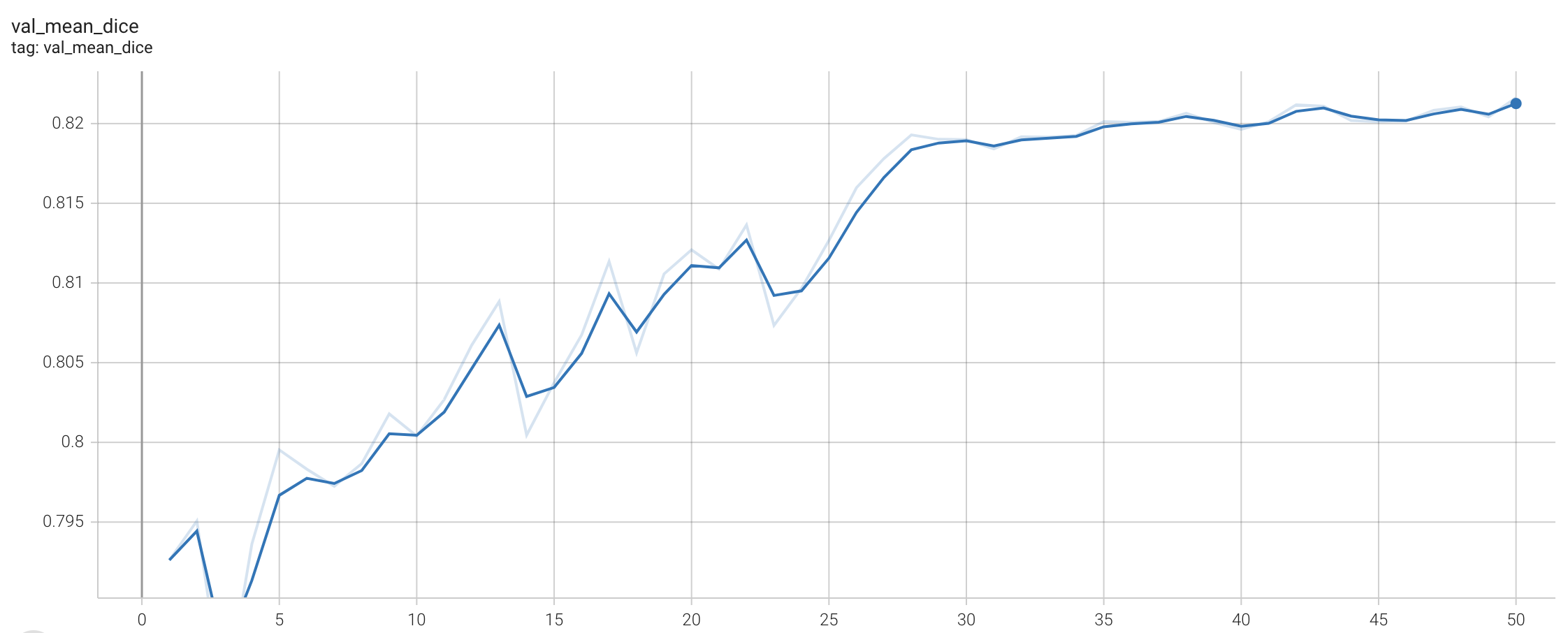 |
|
|
|
#### TensorRT speedup |
|
This bundle supports acceleration with TensorRT. The table below displays the speedup ratios observed on an A100 80G GPU. Please note that 32-bit precision models are benchmarked with tf32 weight format. |
|
|
|
| method | torch_tf32(ms) | torch_amp(ms) | trt_tf32(ms) | trt_fp16(ms) | speedup amp | speedup tf32 | speedup fp16 | amp vs fp16| |
|
| :---: | :---: | :---: | :---: | :---: | :---: | :---: | :---: | :---: | |
|
| model computation | 24.55 | 20.14 | 10.85 | 5.63 | 1.22 | 2.26 | 4.36 | 3.58 | |
|
| end2end | 3451 | 3312 | 1318 | 878 | 1.04 | 2.62 | 3.93 | 3.77 | |
|
|
|
Where: |
|
- `model computation` means the speedup ratio of model's inference with a random input without preprocessing and postprocessing |
|
- `end2end` means run the bundle end-to-end with the TensorRT based model. |
|
- `torch_tf32` and `torch_amp` are for the PyTorch models with or without `amp` mode. |
|
- `trt_tf32` and `trt_fp16` are for the TensorRT based models converted in corresponding precision. |
|
- `speedup amp`, `speedup tf32` and `speedup fp16` are the speedup ratios of corresponding models versus the PyTorch float32 model |
|
- `amp vs fp16` is the speedup ratio between the PyTorch amp model and the TensorRT float16 based model. |
|
|
|
This result is benchmarked under: |
|
- TensorRT: 10.3.0+cuda12.6 |
|
- Torch-TensorRT Version: 2.4.0 |
|
- CPU Architecture: x86-64 |
|
- OS: ubuntu 20.04 |
|
- Python version:3.10.12 |
|
- CUDA version: 12.6 |
|
- GPU models and configuration: A100 80G |
|
|
|
## MONAI Bundle Commands |
|
In addition to the Pythonic APIs, a few command line interfaces (CLI) are provided to interact with the bundle. The CLI supports flexible use cases, such as overriding configs at runtime and predefining arguments in a file. |
|
|
|
For more details usage instructions, visit the [MONAI Bundle Configuration Page](https://docs.monai.io/en/latest/config_syntax.html). |
|
|
|
#### Execute training, the evaluation during the training were evaluated on patches: |
|
Please note that if the default dataset path is not modified with the actual path in the bundle config files, you can also override it by using `--dataset_dir`: |
|
|
|
- Run first stage |
|
``` |
|
python -m monai.bundle run --config_file configs/train.json --stage 0 --dataset_dir <actual dataset path> |
|
``` |
|
|
|
- Run second stage |
|
``` |
|
python -m monai.bundle run --config_file configs/train.json --network_def#freeze_encoder False --stage 1 --dataset_dir <actual dataset path> |
|
``` |
|
|
|
#### Override the `train` config to execute multi-GPU training: |
|
|
|
- Run first stage |
|
``` |
|
torchrun --standalone --nnodes=1 --nproc_per_node=2 -m monai.bundle run --config_file "['configs/train.json','configs/multi_gpu_train.json']" --batch_size 8 --network_def#freeze_encoder True --stage 0 |
|
``` |
|
|
|
- Run second stage |
|
``` |
|
torchrun --standalone --nnodes=1 --nproc_per_node=2 -m monai.bundle run --config_file "['configs/train.json','configs/multi_gpu_train.json']" --batch_size 4 --network_def#freeze_encoder False --stage 1 |
|
``` |
|
|
|
#### Override the `train` config to execute evaluation with the trained model, here we evaluated dice from the whole input instead of the patches: |
|
|
|
``` |
|
python -m monai.bundle run --config_file "['configs/train.json','configs/evaluate.json']" |
|
``` |
|
|
|
#### Execute inference: |
|
|
|
``` |
|
python -m monai.bundle run --config_file configs/inference.json |
|
``` |
|
|
|
#### Execute inference with the TensorRT model: |
|
|
|
``` |
|
python -m monai.bundle run --config_file "['configs/inference.json', 'configs/inference_trt.json']" |
|
``` |
|
|
|
# References |
|
[1] Simon Graham, Quoc Dang Vu, Shan E Ahmed Raza, Ayesha Azam, Yee Wah Tsang, Jin Tae Kwak, Nasir Rajpoot, Hover-Net: Simultaneous segmentation and classification of nuclei in multi-tissue histology images, Medical Image Analysis, 2019 https://doi.org/10.1016/j.media.2019.101563 |
|
|
|
# License |
|
Copyright (c) MONAI Consortium |
|
|
|
Licensed under the Apache License, Version 2.0 (the "License"); |
|
you may not use this file except in compliance with the License. |
|
You may obtain a copy of the License at |
|
|
|
http://www.apache.org/licenses/LICENSE-2.0 |
|
|
|
Unless required by applicable law or agreed to in writing, software |
|
distributed under the License is distributed on an "AS IS" BASIS, |
|
WITHOUT WARRANTIES OR CONDITIONS OF ANY KIND, either express or implied. |
|
See the License for the specific language governing permissions and |
|
limitations under the License. |
|
|